New issue
Have a question about this project? Sign up for a free GitHub account to open an issue and contact its maintainers and the community.
By clicking “Sign up for GitHub”, you agree to our terms of service and privacy statement. We’ll occasionally send you account related emails.
Already on GitHub? Sign in to your account
Divergent wh (width-height) loss #307
Comments
@peterhsu2018 your wh loss is diverging. You should increase your burnin period or decrease |
To answer your other question the |
@glenn-jocher |
@peterhsu2018 The learning rate from the .cfg file is only used if |
Hello, i have a question about the hyperparameters setting in train.py,
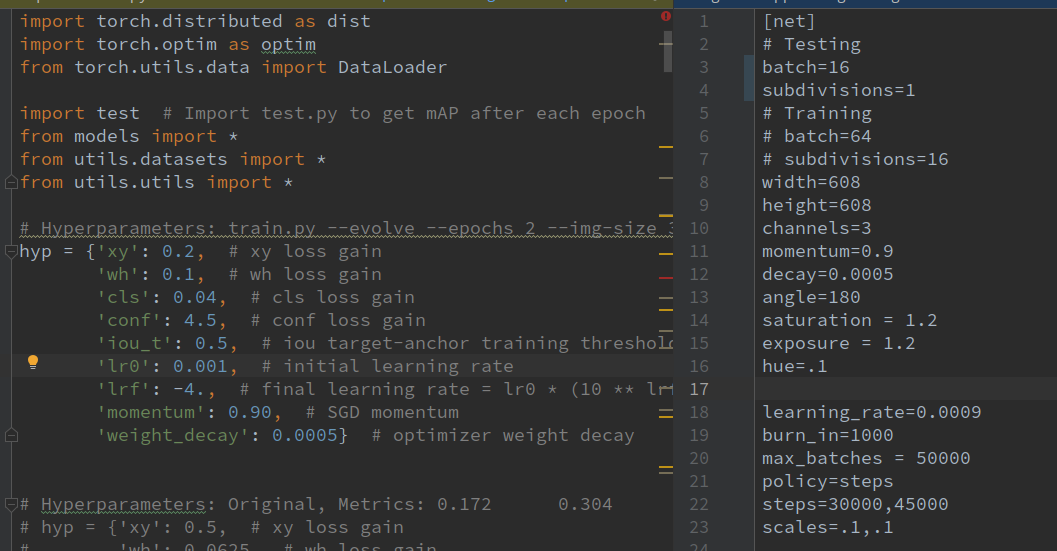
the learning rate here and in my .cfg if different, what's the value will be used?
The text was updated successfully, but these errors were encountered: